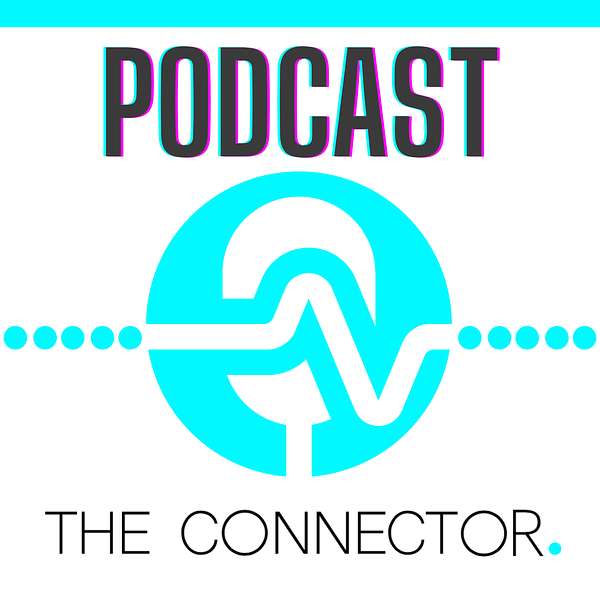
The Connector.
The Connector.
The Connector Podcast - DFS Digital Finance Summit - Advancing AI Risk Management: Jos CEO at Yields on Navigating Regulatory Landscapes and Enhancing AI Reliability Across Sectors
Mathematical modeling expert and Yields co-founder, Jos, joins us to unravel the complexities of AI risk management in our latest podcast episode. Explore Jos's insightful journey from the demanding fields of mathematical modelling to spearheading advanced solutions for AI reliability in production. We dive into how Yields has secured its place as a leader in this space, thanks to accolades like the Risknet Model Validation Award, and how it's successfully transitioned from focusing on investment banking to addressing AI-related challenges across various sectors.
Understanding and managing its risks becomes imperative as AI continues to permeate industries worldwide. In our conversation, Jos shares how Yields guides companies through the evolving regulatory landscapes, such as the EU AI Act, to ensure strong AI governance. With practical examples, including a case from the healthcare sector, Jos illustrates how Yields' software aids organizations in mitigating AI risks. This episode is a treasure trove for fintech enthusiasts and anyone keen on the critical intersection of AI and risk management. Tune in for a compelling discussion that blends technical insight with real-world applications.
Thank you for tuning into our podcast about global trends in the FinTech industry.
Check out our podcast channel.
Learn more about The Connector.
Follow us on LinkedIn.
Cheers
Koen Vanderhoydonk
koen.vanderhoydonk@jointheconnector.com
#FinTech #RegTech #Scaleup #WealthTech
Welcome to the Connector podcast, an ongoing conversation connecting fintechs, banks and regulators worldwide. Join CEO and founder Cohen van der Hooydonk as you learn more about the latest available trends and solutions in the markets.
Speaker 2:Welcome to another podcast, and this one another special one, because it's a collaboration between Fintech Belgium and the Connector. And today I have with me Jos. Somebody I know for a long time has started a company called Yields. So, Jos, welcome to the podcast, and can you please explain who you are and what your company does?
Speaker 3:Thanks, koen, definitely so. My name is Jos Gerardin. I'm one of the founders of Yields, and Yields is a model risk management software company. Now I started at Yields because I've been working in mathematical modeling for a very long time and I noticed that over time, building models, building algorithms, gets gradually more and more easy, but keeping models reliably in production is very hard. Today we see this everywhere. With generative AI, it's very easy to build new chatbots, but to make this chatbot reliable is extremely hard. That's exactly the problem that Yield solves. At Yield, we build software to help to manage the risks of mathematical models going wrong.
Speaker 2:But it actually says award winning. What does that mean?
Speaker 3:Yes, well, it means that we won a few awards.
Speaker 2:A few nice.
Speaker 3:So we have been a winner of the Risknet Model Validation Award for four years in a row there. We have been also mentioned in the data tech 50 shortly shortlist and we have been mentioned on the chartis risk technology top 50 as well, congratulations.
Speaker 2:well, a few years ago, when we met first and we actually wrote an article both in the RECTECH Black Book, yes, I remember vividly. So at the time when we wrote the RECTECH Black Book, you guys were pretty much into investment, world investment banking. Now today you said it a few times I hear a new thing in your speech, which is AI. So how did that came into play?
Speaker 3:yes, good question. So model risk management has been a regulatory requirement for no more than 10, 15, 15 years because, as you know, there have been many accidents with mathematical models over over time.
Speaker 3:Right credit crisis, for instance, was caused by this now today, every company, and banks especially, are using more and more ai. Um. Ai risk is very similar to model risk, because you are using mathematical models in the same way and actually ai systems are now used almost everywhere, so the same problems that we saw 15 years ago with standard statistical mathematical models now appear with AI systems. Moreover, today we also have here in Europe the EU AI Act, which has very similar requirements related to how to mitigate AI risk, how to report about this and how to manage a portfolio of use cases over time. This is exactly the problem that our software solves, and that's why, gradually, more and more, we have more and more customers who use a platform exactly in that context to become AI risk manager and to make sure that AI governance is properly implemented.
Speaker 2:Would you mind sharing how, then, you help to reduce risk on AI?
Speaker 3:Yes, of course. There are typically two aspects to it. First of all, you need to make sure that all the governance related to building AI systems is properly implemented. What this means is that all the data scientists should follow a process that is geared towards better understanding where AI might fail and trying to implement or define controls. I'll give an example, maybe even outside of banking, if you want. Exciting, I will try, because the point is that actually today, we see the same type of risk management issues appearing everywhere, not anymore only in banking. And is that because of the AI element? Yes, of course.
Speaker 2:Yeah, AI goes across the industries.
Speaker 3:You even have toothbrush with AI embedded these days.
Speaker 2:That's why it cleans.
Speaker 3:So well, yeah, well, yes, or you can lose a few teeth if you are part of an AI accident.
Speaker 3:Mistreated by the algorithm Exactly, exactly. No, the example that I was going to give was actually like a serious one. So you know that if you are in hospital, for instance, and they need to measure the oxygen saturation level, they use this sensor with a red light on it. So it has been discovered that actually, that sensor is biased. So if you have people with colored skin, then actually the sensor the algorithm that interprets the sensor data estimates that the oxygen saturation is higher than it actually is, and because of this, during COVID pandemic, for instance, many people of color actually suffered much more, died even because of this issue.
Speaker 3:So this is an example of an AI risk accident. Now how can you mitigate that type of risk? Well, first of all, you need to properly document all your underlying assumptions. In the case of that sensor, it means that actually you have tested this on white skin colored people. You haven't really tested, you haven't really verified that the population was sufficiently representative of the population that you're going to use the sensor with. So you need to actively start thinking about those type of questions. This is what our platform helps you to do to walk you through a process that is designed in such a way that you ask yourself those type of questions first of all, which very much ties into the AI Act, by the way.
Speaker 3:Yes, exactly, it's driven. I mean it's the point of risk management is that you will become compliant to all the relevant regulation along the way. But it's not an a target in itself. It should be a byproduct. The real focus is to mitigate the risk, and so, in the case of the sensor, so you have those more qualitative aspects you need to walk the data scientists through the right process.
Speaker 3:You need to document it properly, such that also the users understand what are all the underlying assumptions. But then, once you have such a sensor, you can also design specific tests to verify that, say, the way in which you are using the algorithm is in line with the assumptions that you made when you designed it. So in this case, for instance, if, say, the sensor would be used on people of color, then because of the signals that are being produced, you can actually detect that you are using the sensor in a place for which it hasn't been designed. And this is like more quantitative tests, and the platform allows you to do both. So to help you operationalizing all the governance and secondly, to then automatically test that actually the all the assumptions that you made initially are still valid and if not, then alarms will be raised, um and like people will be contacted to understand what they need or to to make sure that they focus on that problem and try to solve it.
Speaker 2:What sort of comes to my mind is testing, and think about automation, automation. I think about AI. Do you use AI to solve AI?
Speaker 3:Yes, very good question. We do. However, we are like, first of all, we are using our own approach to mitigate that risk, but we think also very deeply about what are ways of introducing AI such that we do not increase the underlying AI risk. Right, I'll give an example.
Speaker 2:Yes, please.
Speaker 3:When you are trying to mitigate AI risk, an important aspect is documentation, as I mentioned already. Right mitigate AI risk. An important aspect is documentation, as I mentioned already right Now today we all use. Chatgpt or another generative AI.
Speaker 3:Well, yes, for instance, to generate some good questions for a podcast. Now many customers and prospects ask us can we use generative AI to generate documentation? So we always say that we don't do this, and why? Because the process of creating documentation actually helps thinking about what you are actually doing. It has an active element of mitigating risk. However, many people, especially quantitative people like myself they sometimes struggle to create a compelling story or a compelling text when you are creating the documentation. So what we rather do is we use generative AI to help improve the overall quality of the text or to point out that there are some aspects that are not well phrased, but we do not generate the underlying ideas. So in this way, if you do it in this way, then actually you are mitigating the risk because the text that you create can be better understood. However, we are not generating the underlying ideas, which is actually what the model developer has to do.
Speaker 2:No, I get it. I get it. What comes to mind also is when you talk about these models and you've mentioned it before you're a data scientist. One thing I'm thinking of is how do you leverage the data of all the initiative and all the clients that you served? Is there a sense of big data, of of reusing the data for trend analysis or something like that?
Speaker 3:yeah, that's a very good question as well. So obviously many algorithms are being used on massive data sets already right so the platform indeed has to be able to deal with these large amounts of data. However, in most of our use cases, the algorithms are like proper ip over customers yeah, that's what I was thinking and therefore it's very hard and almost never allowed to share data between different customers.
Speaker 3:What we try to do, however, is to share best practices, like model risk management. Ai risk management is not yet a practice that is fully standardized yet. Therefore, every organization uses processes and approaches that are a little bit different. Those processes themselves I mean you can see some that work better than others and we are distilling actively like a set of best practices these we can share clearly across all our customers and also with the community. However, the underlying data we can never do because of IP.
Speaker 2:We're almost at the end of this podcast, but I'm also interested. What yields see in the fields of AI as like the next thing. In terms of testing these algorithms, how do you see the near future evolving?
Speaker 3:Yes, well, we talked a little bit earlier on about generative ai application, especially chatbots and everything related to rags. These are retrieval augmented generation algorithms. This is the most standard approach today to quickly build a generative ai use case. When it comes to testing, there have been a few techniques that have been developed. So far, however, the the community hasn't settled yet on what is the really best practice, and the reason for this is that when you are trying to assess if such an algorithm is working well, there are so many combinations of questions and prompts that you can generate.
Speaker 3:That is very hard. We see that gradually, and especially also thanks to a lot of the input from the financial sector, that some of the techniques become more and more common and we believe that once, say, the community has settled on a good approach there, that we will see a lot more like high risk applications of those racks, like today everyone is hesitating because it's very hard to control. Tomorrow, I think, we'll have like proper techniques to meet it, to to monitor, say, if these rags are working well, and then we'll see again a lot more active use cases. This is, I, a big challenge and a big focus for this year. Another one is how to properly put humans in the loop in a way that you are actually actively mitigating the risk.
Speaker 3:The example I gave earlier about documentation generation versus assessing documentation quality as an example I think, but I will see more and more of those because, just to conclude, um, if you are building a chatbot to generate, say, legal contracts, and those contracts are 300 pages and you can say I'll put a human in the loop by, at the very end, like, ask a human to sign off that this legal text is correct. But maybe the first two times this person is going to be very enthusiastic and read the text. But if you have to do this all day long, after a while I think you'll just sign sign off right. And we need to find better ways of including human inputs without just naively asking at the very end please sign off that this is correct.
Speaker 3:Um I think, we will, as a community, have better um have better processes later on this year to do this, and this again will help to build use cases that can be operated in high stakes context.
Speaker 2:Which is last comment you actually hit exactly on the spot of what was the topic on DFS, which was around how to use human in the process. Well, another topic and that's going to be my last question for you, because you're almost eluding that regulation would help you, Is that correct?
Speaker 3:Yes, well, regulation helps to increase the awareness, especially at sea level, that AI has a huge amount of risk and needs to be actively mitigated. So it's a good driver needs to be actively mitigated. So it's a good driver. Once again, from our point of view, what we try to achieve is to mitigate the risk of using AI and become compliant to the relevant regulation automatically, almost as a byproduct. We never start from the regulation as it is, defining the key requirements, because the regulation is a kind of set of rules that you should ideally apply because that's how the regulator believes you will mitigate risk. But actually actively reducing the risk depends heavily on the context in which you are using an AI system and therefore we kind of turn it around. But it's a very good motivator.
Speaker 3:It increased the visibility and the awareness well, just how can people contact you? Well, they can email me at jos, at yieldsio, or they can, I think, contact you kum and you will be more than happy forward.
Speaker 2:Always happy to forward Perfect. Thanks a lot, Koen. Well, thank you very much for participating. Thank you also to the audience and please stay tuned. More FinTech news to come. Thank you so much.
Speaker 1:Thanks for listening to another episode of the Connector Podcast. To connect and keep up to date with all the latest, head over to wwwjointhekonnectorcom or hit subscribe via your podcast streaming platform.